How an operator can build a big data infrastructure and save $6 million a year from fraud
The telecommunications analytics market is growing by 13.5% annually and will continue to grow until 2030, according to
Valuates Reports. When analyzing large amounts of customer data (or big data), operators discover patterns in user behavior that can be applied for various business purposes, such as improving advertising efficiency, increasing ARPU, retaining customers, and combating fraud. But how can this analytics be implemented to achieve accurate results?
We can exemplify — Eastwind helped build an infrastructure for collecting, processing, and analyzing big data for one of the largest telecommunications companies in the CIS region. With our solution, the operator not only learned to detect fraud and prevent annual financial losses but also increased advertising revenue and reduced subscriber churn.
Key results
Why do operators need to analyze big data?
The average smartphone user spends about 5 hours a day online, according to the
Digital 2023 Global Overview Report. Every action on the device generates online data, whether calling friends, sending messages, searching for products online, or spending time on social media. Information about all these events is sent to the databases of telecom operators that serve millions of subscribers.
Initially, this is "raw" data — information is not structured or processed. Information from different sources is stored in various databases that are often disconnected. To get insights and profit from this array, you need to collect all the data in one place and start searching for patterns using AI/ML technologies.
When you need a platform for working with big data
Companies often deploy a Hadoop cluster to analyze large amounts of unstructured data. This open-source software allows you to store big data and implement all the necessary calculations.
Advantages of the Hadoop ecosystem include free access, many tools, scalability, and costs of support.
Disadvantages of working with Hadoop directly
- The ecosystem is open-source and does not meet all security standards. Hadoop does not clearly divide roles and access rights, and sensitive data may end up in public log files.
- DevOps, data engineers, and the company's analysts must have experience working with all Hadoop tools and know the database languages of all sources. Few such specialists are available, and they are expensive.
- The system is vulnerable. If a task calculation error occurs or an incompatible library is installed, the entire analytical algorithm may stop working.
The Eastwind DataFlow platform, which we implemented for our client, allows us to build a big data infrastructure using Hadoop's advantages while eliminating all its associated disadvantages.
Learn more about DataFlow
Project format
The work was carried out in collaboration with the operator and the integrator partner. At the start of the project, we set the following tasks for implementation:
1. Consolidate all data and organize its storage. This step became the basis for big data analysis and the implementation of AI/ML technologies.
2. Implement predictive analytics in the following areas:
— Fraud prevention
— Preventing subscriber churn
— Effectiveness of cross-sell and upsell campaigns for key products
The client also wanted to develop the project independently in the future by integrating new data sources and creating and refining ML models. During the project, the operator formed a team of full-time data analysts — Eastwind specialists also helped them with this.
Timeline
Step 1. Integration with data sources
Before launching the project, many operators' systems worked independently. What we had at the start:
— Technological and geographical fragmentation of data sources (17 territorial units)
— Complex IT landscape, which included dozens of platforms and solutions from different vendors
— Non-relational and specific databases
After our team deployed and configured a Hadoop cluster of 24 hosts with a total capacity of 1.8 PB, we began integrating sources into a single data lake.
To fill the data lake, Eastwind provided the connection of the necessary data sources, the partner-integrator filled the data on the cluster and described the regular import using DataFlow, and the operator controlled the data integration on the source side and agreed on the methods of loading data and their description.
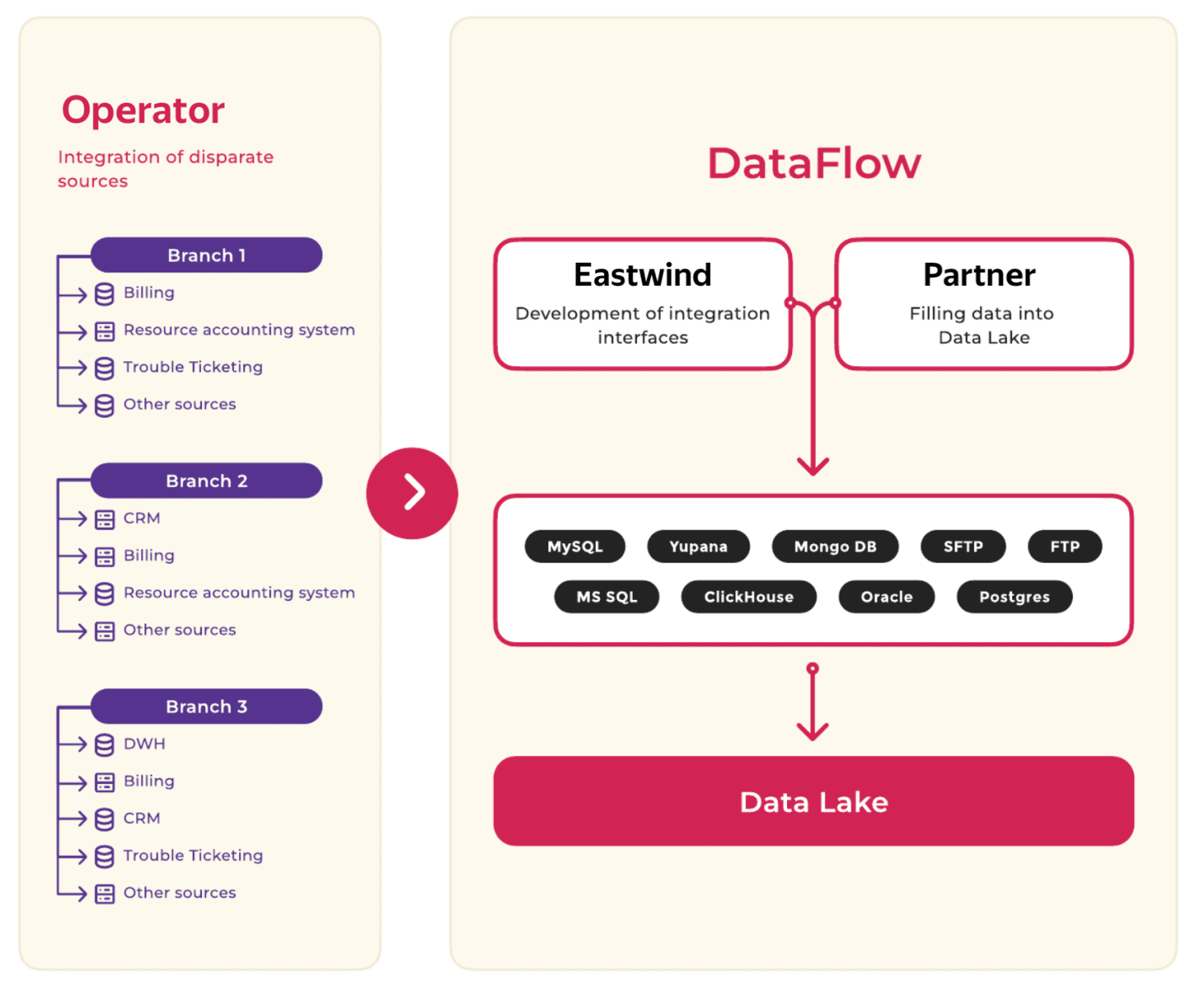
First, the project team integrated data from the operator's internal information systems, including corporate storage, billing, resource accounting, and reporting systems. Then, we connected third-party sources, such as the state open data portal and others.
Step 2. Implementation of predictive analytics
When the project team integrated enough data sources, we built a business process for testing hypotheses using ML models.
The work was carried out in three areas: anti-fraud, subscriber churn prediction, and building an NPTB (Next Product to Buy) model.
Anti-fraud models
The objectives in this area were to reduce the operator's financial losses, mitigate reputational risks, and prevent subscriber complaints about fraud.
At the hypothesis generation stage, the project team identified 20+ different types of fraud and developed 30+ analytical models. Based on the results of the first check, four of them were able to detect the following fraudulent cases effectively:
Implementing analytical models to combat fraud has reduced the level of doubtful accounts receivable, eliminating fraudulent charges and the risk of legal costs.
Director of the operator's data analytics department
$1,100,000 per year
prevented losses — the effect of implementing four cases in the first year, calculated by the company based on historical data on fraud for previous years |
$6,000,000 per year
estimate of operator's prevented losses after implementing another 40 fraud detection cases, which analysts generated and configured after the launch of the entire infrastructure |
Predicting subscriber churn
This model aims to detect customers who are likely to change their operator in advance. One of the many indicators of future churn is subscriber dissatisfaction with service, tariffs, or connection quality. Therefore, when developing the churn model, the project team automated the loading of Excel tables with records of subscriber requests via WhatsApp, Telegram, and social networks into DataFlow.
The analytical algorithm was also adapted to all 17 regions of the customer's presence, each could have its reasons for changing the operator (for example, local competitors).
This approach allowed us to gradually improve the forecast results. At the beginning of the work, the accuracy of the model was 0.12, and the precision was 0.13. Less than a year later, the accuracy increased fourfold — 0.50; precision doubled — 0.30. This means that the model correctly divided 50% of the subscriber base into those who were leaving and those who were not, while detecting 30% of all customers who were going to leave.
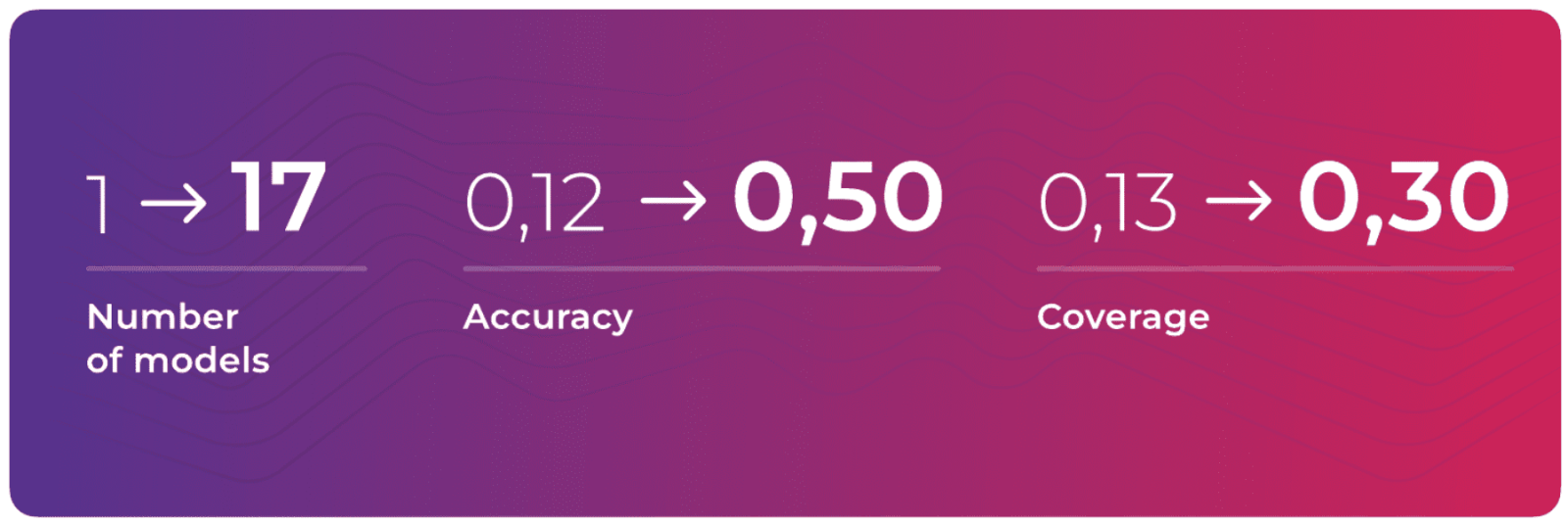
In the second year of the model's operation, we set a goal of achieving 70% accuracy and 50% precision. But even now, a fundamental difference in working with the client base is visible, compared to the period before the deployment of the big data infrastructure. Previously, we dealt with subscribers who came to the office with the goal of canceling the service, and only after that we tried to convince them to stay. Now, we retain 30% of such subscribers preventively. This significantly increases the chances of convincing those who are churning and reduces the costs of their retention.
Director of the operator's data analytics department
Models Next Product to Buy
Initially, the project team implemented NPTB models for two core products — the operator's main tariff plans. The specialists created ML algorithms that analyzed the profiles of subscribers who had been using the selected products for a long time. The models then examined the entire customer base and created lists of users who were most likely to switch to the promoted tariff.
The operator passed these lists to call center employees to make calls offering to update the tariff plan. Statistics on subscriber purchases and refusals were used to further train the predictive model. After achieving a positive effect on the main tariffs, additional products were launched and six more predictive models were created for cross- and upsell sales.
$ 2,280,000
generated additional income from cross- and upsell sales based on Next Product to Buy models in the first year of the project |
General results and development of the project
With the implementation of DataFlow, the operator received a ready-made infrastructure for analyzing customer data. Now it can independently connect any sources of information, create predictive models, and quickly launch them into production. The client also began using big data analytics to evaluate the effectiveness of all business activities. For example, he planned to implement cases for predicting equipment failure in order to take maintenance measures in advance. What else was included in the development plans:
- Connect 15 new data sources, including unstructured text dialogues from operator support chats and NPS survey results
- Launch external monetization cases, for example, determine the most advantageous location of sale points
- Upgrade the CVM platform to enable more flexible marketing campaigns
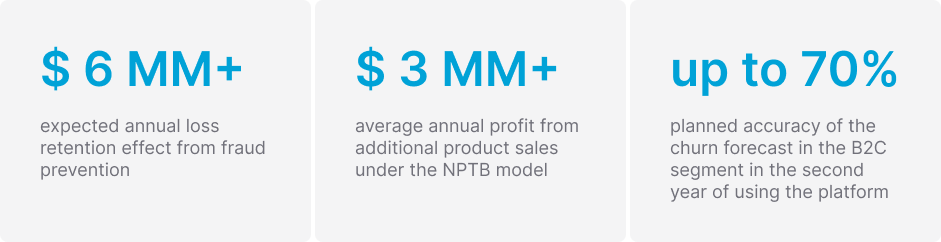
Big data capabilities with DataFlow
The Eastwind platform allows you to implement the entire process of working with data in one window:
— Connect new data sources
— Select the necessary data samples and conduct research
— Build analytical models and quickly launch them into production
— Export data to platform consumers
— Monitor calculations, detect errors, and track the quality of models
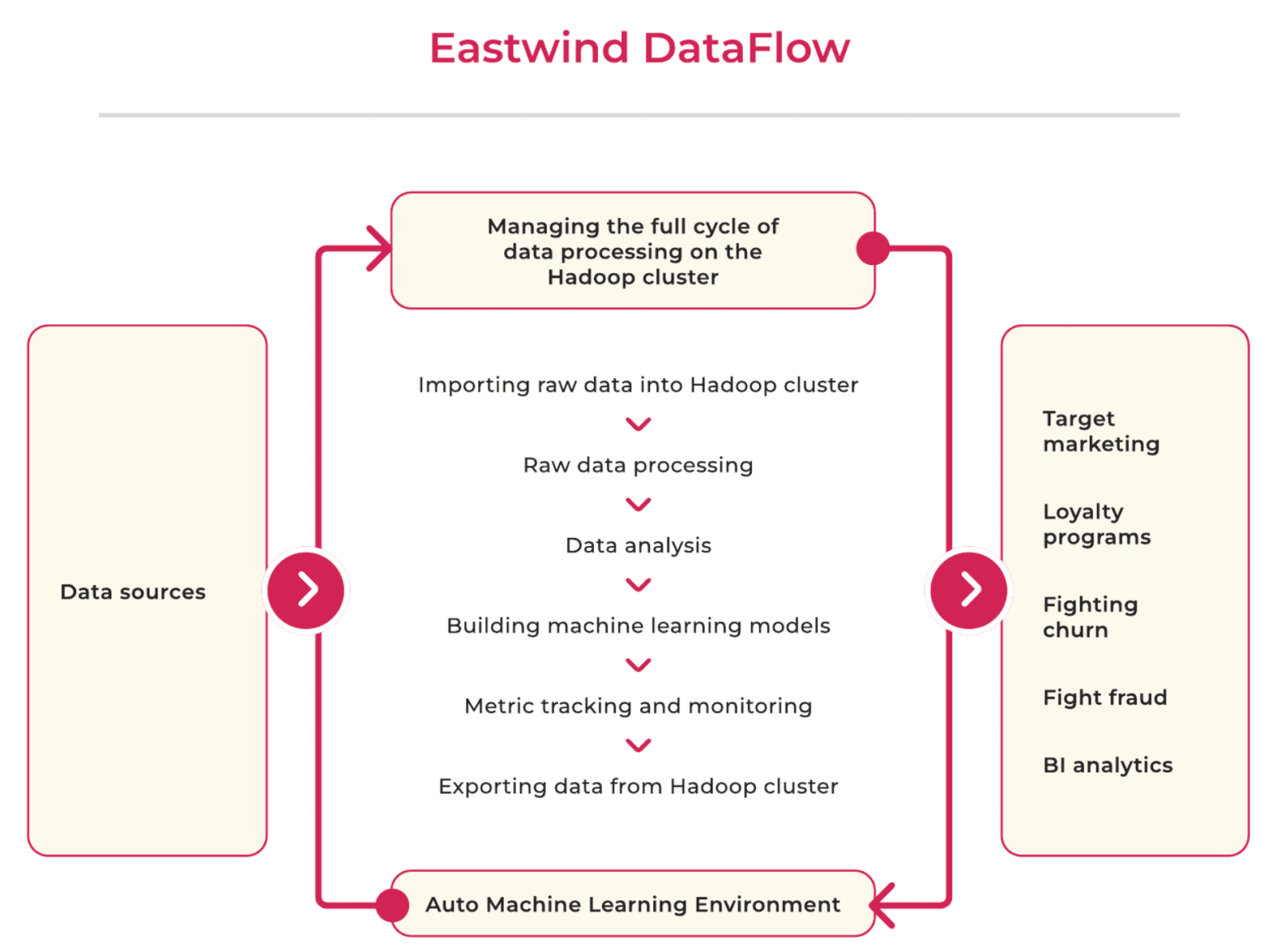
The platform has pre-configured interfaces for working with most databases, which simplifies and speeds up the work process and reduces the solution's user requirements. DataFlow also provides a high level of data security, with a role-based access model, corporate authentication, and hiding all sensitive information transmitted in the Hadoop environment.