Scoring Case Forum 2017 Looks at Borrower Evaluation Trends and Banking Life Hacks
27 july 2017
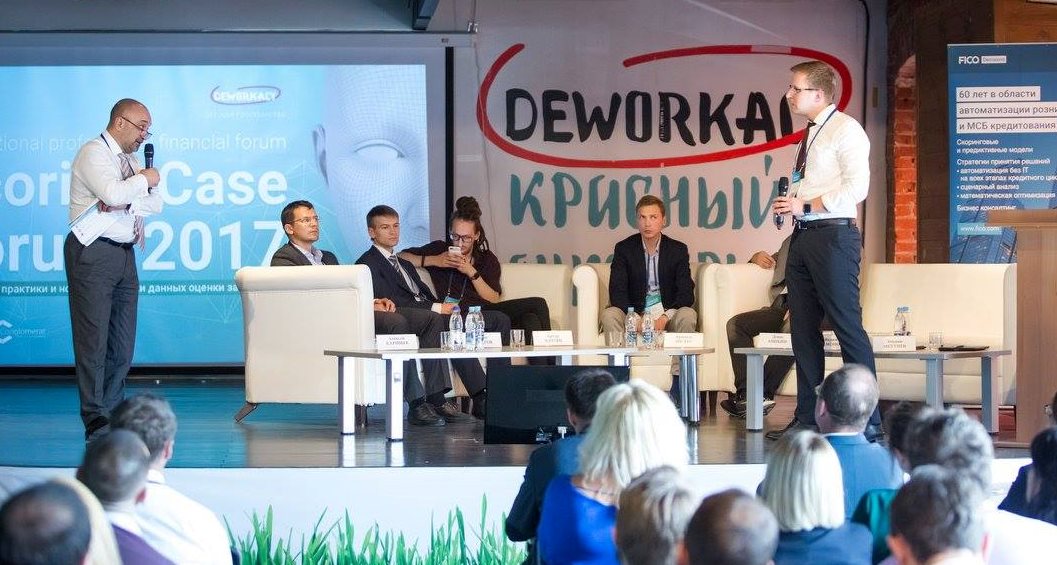
July 5, analysts and financial businesses from micro-loan companies to the largest banks met in Deworkacy business space in Moscow. The forum was dedicated to new Big Data technologies –unconventional scoring methods that take into account not only the client's solvency, but also its psychotype. We learned who will be given credit under the new rules, and who will be denied, and asked what our Big Data experts think about this.
Financial market tends to transform rapidly, which cannot always be said about banking scoring methods. Alexey Chalenko, CEO of 7seconds analytics service, gave a blunt yet illustrative description of the ideal bank borrower: "It’s an old sick married doctor from Tajikistan." This is benchmark for the traditional machine algorithms that decide whether to give the client a loan or not. Banks have no idea how to work with students, generations X or Y, foreigners or pensioners, says Elena Koneva, FICO’s scoring director. Such people are denied loans, though in fact they could be reliable and solvent borrowers.
This reveals the imperfection of the old scoring models designed to evaluate three parameters: fraud probability, ability to pay, and willingness to pay. Today, most banks are focused on the second characteristic: they want to know the length of the client's experience, their salary and address. But the world is changing rapidly: a freer attitude to work and place of residence are becoming ever more commonplace. It is willingness to pay that becomes the main characteristic in the evaluation of a potential client – their level of responsibility, and their lifestyle. When estimating this parameter, new sources of data can help: from payment systems to social networks and mobile operators.
The second global problem is how to detect fraudsters promptly, amid the increasingly flexible scoring systems. Loan service MoneyMan suggests a fraud scoring system be put at the beginning of the customer evaluation chain. In 2017, data scientists ID Finance have launched a new fraud detection model for the company, which helped to reduce the cost of loan by 25%. Irina Horoshko, Risk Director at MoneyMan, says the system uses 15 new data sources and evaluates applications in a fundamentally new way. For example, it times how fast a client fills in their full name: a normal person takes about 10 seconds to do this. If a user spends considerably more time on the action – they are probably a scam, and the application will be rechecked.
Another question we asked was what data for accurate borrower assessment are in shortage now. Maria Weichmann, CEO of Scorista noted that in China, the financial sector scoring has successfully used data on bank card transactions and on the use of telecommunications services. In Russia, this area is also actively developing, supported by large operators and vendors. The latter help to create, configure and test analytical platforms on the spot - that is, directly at the data source. Telcos provide scoring models based on their data to banks as well as use them for their own purposes. Have you ever stopped to think that subscribers often borrow? The ability to talk when your balance is negative, and use deferred payment – these are all loans that users can obtain from operators. The trends here are the same: new approaches to borrower assessment and anti-fraud protection.
Checked by Eastwind: It works!
We implemented a new scoring model for subscriber evaluation as part of a project for one of the largest CIS telecom operators. We used our EW Social Analytics platform to generate 300 subscriber parameters. Apart from information pertaining to payments, the list included interests, community level, geo location, and much more. The model assessed credit risks in real time and determined which users should not be allowed to use the ‘deferred payment’ service. After testing, the results were compared against the statistics on loans the operator had issued using traditional valuation. With the new Eastwind model, fewer clients were allowed to use the service. At the same time, we managed to reduce three times the percentage of subscribers who did not return their debt. The result: the operator’s actual revenue has grown by 42%.
As we see, new scoring methods should be comprehensive and cover more parameters in order to work effectively. Today’s analytical systems allow you to study the borrower’s personality and behavior as well as their solvency. The advance and ubiquitous implementation of these technologies in finance and other areas will allow a more accurate customer assessment, which means more right decisions: negative for potential debtors and fraudsters, and positive for those who are willing and going to pay.
More News
Financial market tends to transform rapidly, which cannot always be said about banking scoring methods. Alexey Chalenko, CEO of 7seconds analytics service, gave a blunt yet illustrative description of the ideal bank borrower: "It’s an old sick married doctor from Tajikistan." This is benchmark for the traditional machine algorithms that decide whether to give the client a loan or not. Banks have no idea how to work with students, generations X or Y, foreigners or pensioners, says Elena Koneva, FICO’s scoring director. Such people are denied loans, though in fact they could be reliable and solvent borrowers.
This reveals the imperfection of the old scoring models designed to evaluate three parameters: fraud probability, ability to pay, and willingness to pay. Today, most banks are focused on the second characteristic: they want to know the length of the client's experience, their salary and address. But the world is changing rapidly: a freer attitude to work and place of residence are becoming ever more commonplace. It is willingness to pay that becomes the main characteristic in the evaluation of a potential client – their level of responsibility, and their lifestyle. When estimating this parameter, new sources of data can help: from payment systems to social networks and mobile operators.
The second global problem is how to detect fraudsters promptly, amid the increasingly flexible scoring systems. Loan service MoneyMan suggests a fraud scoring system be put at the beginning of the customer evaluation chain. In 2017, data scientists ID Finance have launched a new fraud detection model for the company, which helped to reduce the cost of loan by 25%. Irina Horoshko, Risk Director at MoneyMan, says the system uses 15 new data sources and evaluates applications in a fundamentally new way. For example, it times how fast a client fills in their full name: a normal person takes about 10 seconds to do this. If a user spends considerably more time on the action – they are probably a scam, and the application will be rechecked.
Another question we asked was what data for accurate borrower assessment are in shortage now. Maria Weichmann, CEO of Scorista noted that in China, the financial sector scoring has successfully used data on bank card transactions and on the use of telecommunications services. In Russia, this area is also actively developing, supported by large operators and vendors. The latter help to create, configure and test analytical platforms on the spot - that is, directly at the data source. Telcos provide scoring models based on their data to banks as well as use them for their own purposes. Have you ever stopped to think that subscribers often borrow? The ability to talk when your balance is negative, and use deferred payment – these are all loans that users can obtain from operators. The trends here are the same: new approaches to borrower assessment and anti-fraud protection.
Checked by Eastwind: It works!
We implemented a new scoring model for subscriber evaluation as part of a project for one of the largest CIS telecom operators. We used our EW Social Analytics platform to generate 300 subscriber parameters. Apart from information pertaining to payments, the list included interests, community level, geo location, and much more. The model assessed credit risks in real time and determined which users should not be allowed to use the ‘deferred payment’ service. After testing, the results were compared against the statistics on loans the operator had issued using traditional valuation. With the new Eastwind model, fewer clients were allowed to use the service. At the same time, we managed to reduce three times the percentage of subscribers who did not return their debt. The result: the operator’s actual revenue has grown by 42%.
As we see, new scoring methods should be comprehensive and cover more parameters in order to work effectively. Today’s analytical systems allow you to study the borrower’s personality and behavior as well as their solvency. The advance and ubiquitous implementation of these technologies in finance and other areas will allow a more accurate customer assessment, which means more right decisions: negative for potential debtors and fraudsters, and positive for those who are willing and going to pay.